


In the 2-D case, the Wiener and homomorphic filters have very effective realization when images are described in tensor representation as an unique set of 1-D image-signals and the 2-D discrete Fourier transform (DFT) is split into a set of 1-D DFTs of image-signals. Robustness of the optimal filters is also described and examples of image restoration are given. This constraint requires the PSD of the filtered signal to be equal to the PSD of the uncorrupted signal that it estimates. The geometric mean filter for, which is called the homomorphic filter, is the optimal linear filter for the model under power-spectral-density (PSD) equalization. For k=1 and k=0, the geometric-mean filter gives the inverse filter and the Wiener filter for the model, respectively. These filters are used to provide frequency-based filtering that mitigates the noise suppression of the optimal-linear Wiener filter in the blurred-signal-plus-noise model. The Wiener filters which are the best linear filters are described, as well as the geometric-mean filters that compose a family of filters indexed by a parameter k varying between 0 and 1. In this paper we analyze the methods of optimal restoration of signals and images from the degradations that are defined by the additive noise and blurring effects. Moreover, a global filter can be designed that tends to maintain performance across states, albeit, at the cost of some increase in error relative to specific states. Then, based on these prior distributions determining the design conditions, we can evaluate filter performance across the various states.

This results in the robustness problem: how well does a filter designed for one environment perform in a changed environment? This problem is studied by considering the ideal and observed images to be determined by distributions whose parameters are random and possess prior distributions. A second problem is that a filter must be applied in imaging environments different from the one in which it is designed. This paper discusses two types of prior information that can facilitate design for large windows: design by adapting a given (prior) filter known to work fairly well and Bayesian design resulting from assuming the conditional probabilities determining the optimal filter satisfy prior distributions reflecting the possible states of nature. For large windows, design is hampered by the exponentially growing number of window observations. This filter is typically designed from pairs of ideal and observation images, and the filter used in practice is the resulting estimate of the optimal filter, not the optimal filter itself. The optimal binary window filter is the binary conditional expectation of the pixel value in the ideal image given the set of observations in the window. Figure 8 shows a noisy realization of the gothic 7a is 0.030 and MAEs for the filtered images computed over large realizations are 0.0123, 0.0129, and 0.0133 for p p, p0.06, and p0.01, respectively.
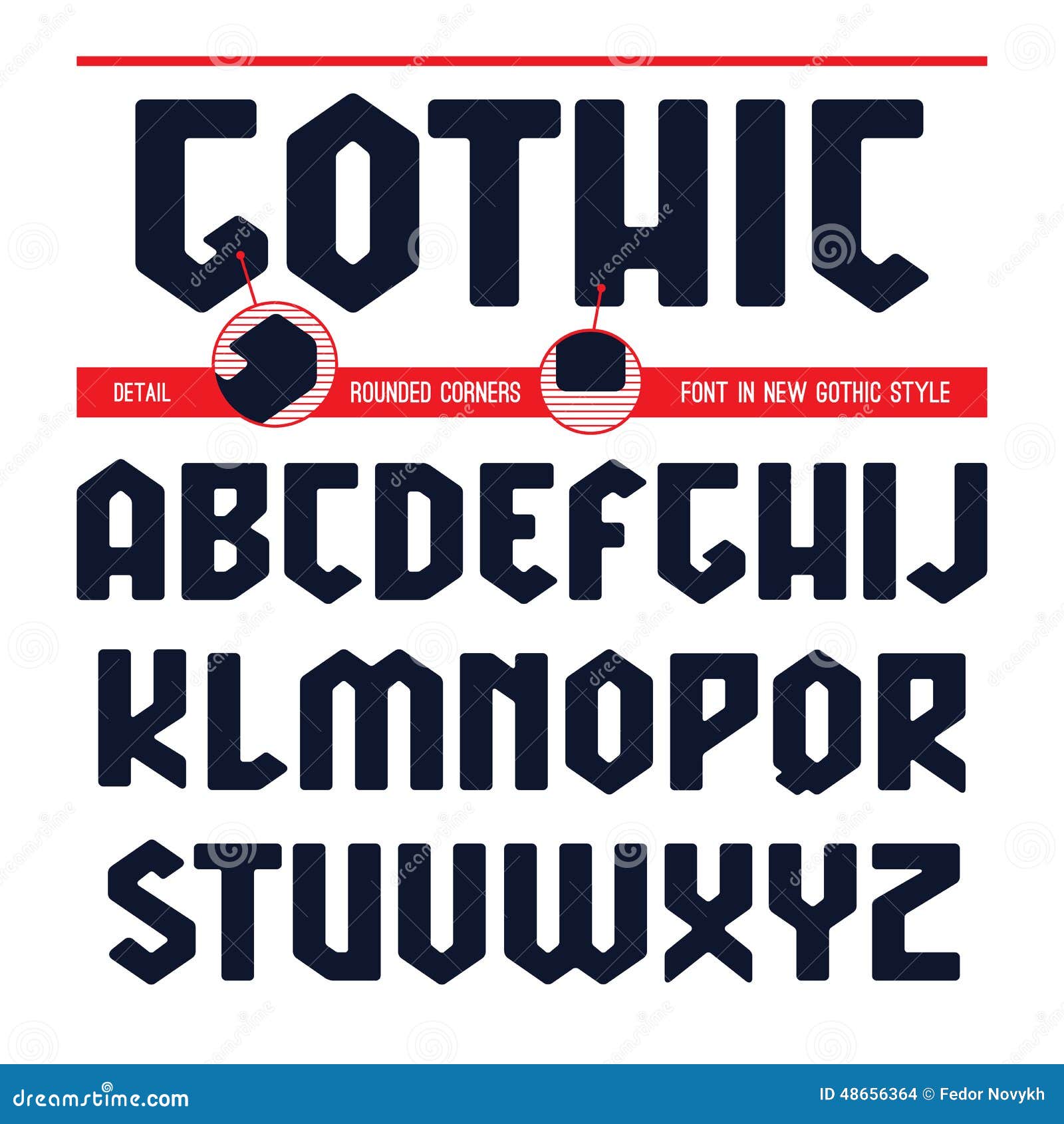
7 shows a noisy realization of the triplex font for p0.03, and the results of filtering the realization by the optimal filters for pp, p0.06, and p0.01. To illustrate the difference between triplex and gothic fonts, Fig. The robustness surfaces for triplex and sans serif fonts are similar, and optimal filters for these fonts show more robustness than filters for gothic fonts when filtering independent sparse noise. For analytic computation of the robustness surface p can go to 1/9 however, we use range 0,1/11 because for simulated cases the maximum cannot be achieved. 4 through 6, respectively, where p values run from 1/11 out to 0 and where the p design axis is to the left. Robustness surfaces for triplex, sans serif, and gothic fonts are shown in Figs. 3 shows sparse-noise-degraded versions of these realizations for two different intensities, p0.030 for Figs. Shows realizations of triplex, sans serif, and gothic fonts, and Fig.
